Policy Evaluation Flashcards
Identifying assumptions - RCT
- treatment and control groups are on average the same
- on observable and unobservable characteristics; SUTVA
Identifying assumptions - regression
- treatment is as good as randomly assigned conditional on observables (CIA)
Identifying assumptions - IV, RD
- relevance; independence; exclusion
identifying assumptions - DiD
- common trends; no compositional changes; homogenous treatment effect
- Two identifying assumptions for DiD:
- No compositional changes between two groups: e.g. in the minimum wage example if some restaurants go bankrupt, then left with surviving restaurants
- Common trend, i.e. time effects between groups are the same; cannot prove it, have to assume
- What you can look into is how similar trends of two groups were before time 0; if they are similar, indicates that they might be similar in observed period, but cannot prove since we never observe red line in graph on p. 5
- Be cautious of level vs. log: if common trend in levels, no common trend in logs unless they are the same line (look into this more)
identifying assumptions - bounds
- monotone treatment response; monotone treatment selection; monotone instrumental variable
stable unit treatment value assumption (SUTVA)
- definition: The potential outcomes for any unit do not vary with the treatments assigned to other units, and, for each unit, there are no different forms or versions of each treatment level, which lead to different potential outcomes. SUTVA requires that the response of a particular unit depends only on the treatment to which he himself was assigned, not the treatments of others around him.
- example: would be violated for treatments of inectious diseases as my outcome (disease or not) depends on treatment of others (if lots treated, less likely to get disease)
- No interference. Often not credible in classroom/school setting; therefore randomize at level of schools classes/schools instead of students
- If maybe spillover between units in different treatment groups, can change their unit of analysis. Students assigned to tutoring program might interact with other students in their school who were not assigned to the tutoring program and influence their grades. To enable causal inference, the analysis might be completed at the school level rather than the individual level. SUTVA would then require no interference across schools, a more plausible assumption than no interference across students. However, generally entails a sharp reduction in sample size. Also changes question that we can answer: no longer learn about the performance of individual students, but of schools.
- No hidden variations of treatments. For example: it shouldn’t matter whether treatment was assigned or chosen
Randomized trials - advantages
- Random assignment ) Di is independent from characteristics of participants
- justifies OLS without control variables (i.e. E (ui|Di) = 0)
- Randomization can be based on (pre-treatment) covariates Xi
- justifies OLS with control variables Xi (i.e. E (ui|Di, Xi) = E (ui|Xi))
- Multiple treatment groups/levels of Di are possible
Randomized trials - statistical inference and power
- We never observe the underlying truth, only the data from our sample
- Four possible cases:
- False positive: we find a statistically significant effect even though the true effect is zero (Type I error)
- True zero: we fail to reject H0 and there truly is no effect
- False zero: we fail to reject H0 even though the true effect is not zero (Type II error)
- True positive: reject H0 and there truly is an effect. This is the power, the probability that we will not make a type II error: (1 )
- power is typically set at 0.8 or 0.9
Randomized trials - determinants of power
- likely not on exam
- Effect size
- what chance of finding a statistically significant effect if the true effect size is beta*?
- given n, what is minimum level of true effect at which we have enough power to reject H0?
- Residual variance
- including control variables (past outcomes) often reduces residual variance - the width of the bell curves
- Sample size
- also affects the width of bell curves
- Statistical significance
- increasing (from say 5% to 10%) increases the power
- Allocation ratio
- fraction of sample that is assigned to treatment
- Clustering
- individuals versus schools/classes as unit of randomization

Minimum detectable effect size
likely not on exam

What we need for a power calculation
- likely not on exam
- Desired power: 80 percent, 90 percent …
- MDE size: hardest part, matter of judgement; not a goal or prediction
- using “standard” effect sizes based on a large number of experiments on education; 0.2 is small but respectable, 0.4 is large
- compare various MDE sizes to those of interventions with similar objectives (aiming at same outcome)
- what effect size would make the program cost-effective?
- Number of clusters: if cost is not an issue, maximize J, otherwise balance J and g relative to the costs
- Allocation fractions: pT/pC = squareroot(cc/ct) (assumes given budget for program+evaluation; c per person cost)
- Estimate of the residual variance (historical data, pilot)
- Estimate of the intracluster correlation (historical data, pilot)
how a small portion of false positives can be very misleading
- Of hypotheses interesting enough to test, perhaps one in ten will be true. So imagine tests on 1,000 hypotheses, 100 of which are true.
- The tests have a false positive rate of 5%. That means they produce 45 false positives (5% of 900). They have a power of 0.8, so they confirm only 80 of the true hypotheses, producing 20 false negatives.
- Not knowing what is false and what is not, the reseacher sees 125 hypotheses as true, 45 of which are not. The negative results are much more reliable–but less likely to be published.
three types of peer effects
- Endogenous interactions
- direct effect from behavior of others on own behavior
- Contextual interactions
- the exogenous characteristics of others affect ones’ behavior
- Correlated effects
- shared environment or characteristics which are unobserved
- why make these distinctions? Separate peer effects (endogenous/contextual interactions) from confounders (correlated effects); implications for spillovers:
- Example: suppose we do extra tutoring
- with endogenous effects other students will indirectly benefit
- not the case with contextual interactions and/or correlated effects
- Example: suppose we do extra tutoring
three problems with identifying peer effects
- The reflection/simultaneity problem:
- Do peers affect respondent, or does respondent affect peers?
- The self-selection problem:
- Peers (including respondents) select themselves on similar characteristics
- The correlated unobservables problem
- Are effects driven by behavior or by unobserved characteristics that are correlated with it.
- It is not always clear how to define a peer group.
Randomized trials 1 - Duflo, Dupas, Kremer: Peer effects, teacher incentives, and the impact of tracking: Evidence from a randomized evaluation in Kenya
Experiment
- Randomized experiment in Kenya
- 121 primary schools with one 1st grade class, extra teacher for 2 sections
- Two random treatments
- 60 schools split group randomly in two
- 61 schools track by first term grades
- Estimate average effect of tracking
- Estimate effect of tracking for the median student
Results
- Tracking is beneficial for students above the median
- Tracking is beneficial for students below the median
- For the median student, it doesn’t matter whether s/he is placed in the upper or the lower track
- What type of production technology and incentive structure could generate these results?
- Linear-in-means (+) can only explain 1, not 2 and 3
- Linear-in-means (+) and linear-in-spread (–) can explain 1 and 2, but not 3.
Issues
- results violate linear-in-means model
- both students at bottom and top get higher scores with tracking - would only expect that for students in class with lower ppers
- at middle, continuous, i.e. doesn’t matter if average and placed in higher vs lower groups
- lack of difference might be explained by role of teacher - they focus on better-performing students and base level of study on higher students, so for avg student in to group leve of study is much further from their ability than for below average guy who is in power group and there is a high performer
Randomized trials 2 - Miguel, Edward and Kremer: Worms: identifying impacts on education and health in the presence of treatment externalities
- Results only become insignficant if you do three out of four things at the same time (blogs by Blattman and Ozler):
- divide data into two smaller samples (year 1 and year 2)
- ignore spillovers
- average outcomes at the school level
- redefine treatment periods to match calendar years
- ATE seemed low, so thought pills were ineffective, but that was mostly due to externalities–>get people not treated benefitted from treatment of others
Regression - OVB
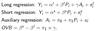
Regression - good vs bad controls
- Some variables are bad controls and should not be included in a regression model even if inclusion changes the coefficient of interest
- Bad controls are variables that are themselves outcome variables
- Good controls are variables that have been fixed at the time the regressor of interest was determined
- Example: Suppose we are interested in the effects of a college degree on earnings and that people can work in one of two occupations, white collar and blue collar
- A college degree clearly opens the door to higher-paying white collar jobs. Should occupation therefore be seen as an omitted variable in a regression of wages on schooling?
- Lets look at the effect of college on wages for those within an occupation, say white collar only
- The problem is that if college affects occupation, comparisons of wages by college degree status within an occupation are no longer apples-to-apples, even if college degree completion is randomly assigned
- Bad control means that a comparison of earnings conditional on occupation does not have a causal interpretation
quantile regression
left out, check if necessary
IV - reasons to use IV
use if cov(u,x)/=0, i.e. endogeneity

IV - how it works
- We want to split x into two parts:
- part that is correlated with the error term (causing E[ui|xi]/=0)
- part that is uncorrelated with the error term
- In order to isolate the second part use a variable z with the following properties:
- exogeneity
- relevance
IV - one endogeneous regressor, one instrument

IV - reduced form
follows from substituting FS into structural equation
IV - Wald estimator (one endogenous regressor, one instrument)
- Wald estimator is IV estimate (transparent version of it)
- E(Y|Z=1) – E(Y|Z=0) (Z is the instrument) – this is the reduced form – divided by E(X|Z=1)-E(X|Z=0) (which is the FS)

IV - insutrment exogeneity and Hausman test
- for IV relevance, can simply take F-stat of FS coeffcient of Z on X; rule of thumb: F>10
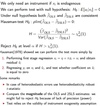
IV - overidentifying restrictions test
- exam question!
- Overidentification restriction test: assuming you have multiple instruments, testing that IV estimate that we get is the same for one instrument as for the other or for any combination of the instruments; so basically testing if on instrument is valid given (assuming) that the other instrument is valid
- exam question: should be able to explain in exam why this is nonsense (i.e. look up why overidentification restriction test is not accurate, working etc.)
- One problem: strong assumption that one is valid
- Answer has to do with Wald estimate / IV: add a 1 to Z in Wald estimator (i.e. Z1); if for Z1 have certain LATE estimate, no reason to assume that instruments effect the exact same groups in exactly the same way (only if that was the case would the Wald estimates be the same? This in parentheses is my addition, so check); only makes sense if we believe in homogeneous treatment effects

IV - monotonicity assumption in heterogeneous effects setup
- all those affected by the instrument are affected in the same way
- If monotonicity assumption violated very problematic; e.g. have FS of 0.3, assume this is share of compliers, but if have defiers, could be 0.31-0.01 (would not be that problematic), but if 0.6-0.3 then problematic
- Arguments for why monotonicity should hold in papers: small number of defiers
- in example of the effect of military service on earnings (Angrist, 1990):
- Monotonicity implies that there is no one who would have served in military if given a high lottery number, but not if given a low lottery number
- Assumption would be violated if someone, who would have volunteered for the Navy when not at risk of being drafted (high lottery number), would have chosen to avoid military service altogether when at risk of being drafted (low lottery number)