Econometrics Week - Time Series Flashcards
first difference
change in value of Y between period t-1 and period t is Yt-Yt-1
lagged value
the value of Y in the previous period relative to hte current period, t: Yt-1
why do we often use logs in economic time series?
- many economic series exhibit growth that is approx. exponential; that is, over the long run, the series tends to grow by a certain percentage per yer on average. This implies that the log of the series grows approx. linearly
- another reason is that the sd of many economic time series is approx. proportional to its level; that is, the sd is well expressed as a percentage of the level of the series- This implies that the sd of the log of the series is approx. constant
- In either case, it is useful to transform the series so that changes in the transformed series are proportional changes in the origina series, and this is acihieved by log
autocorrelation and autocovariance
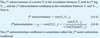
stationarity
- background: time series forecasts use data on the past to forecast the future. doiig so presumes that the future is similar to the past in the sense that the correlations, and more generally the distributions of the data in the future will be like they were in the past. If this wasn’t true, then historical relationships would likely not be reliable forecasts of the future
- definition: probability distribution of the time series variable does not change over time. Under the assumption of stationarity, regression models estimated using past data can be used to forecast future values
- In other words: stationarity holds when the joint distribution of (Ys+1,…,Ys+T) does not depend on s
- In other words, (Y1, Y2,…,YT) are identically distributed, however, they are not necessarily independent!
- reasons for non-stationarity:
- unconditional mean might have a trend, e..g US GDP has a persistent upwrad trend, reflecting long-term economic growth
- population regression coefficients change at a given point in time
mean squared forecast error
- because forecast errors are inevitable (future is unknown), aim is not to eliminate errors but to make them as small as possible–>MSFE = E[YT+1 - YhatT+1|T)2] (the T+1|T means that the forecast is of the value of Y at time T+1 made using data up until T)
- the MSFE is the expected value of the square of the forecast error
- the root mean squared forceast error (RMSFE) is the square root of the MSFE. Same units as Y
- if the forecase is unbiased, forecast errors have a mean of 0 and the RMSFE is the sd of the out-of-sample forecast
- large forecast erors often more costly than small ones; series of small forecast errors often only causes minor prolem, but big one can call entire forecast into question
- MSFE incorporates two sources of randomness
- randomness of future value, YT+1
- randomness arising from estimating forecast model
- by adding and subtracting muy, if YT+1 is uncorrelated with muhaty, the MSFE can be written as MSFE = E[YT+1-muy)2] + E[muhaty-muy)2]; the first expression is the error the forecaster would make if the population mean were known; this captures the random future fluctuations in YT+1 around the population mean; the second term is the additional error made because the population mean is unknown, so forecaster must estimate it
autoregression
expresses the conditional mean of a time series variable Yt as a linear funciton of its own lagged values. A first-order autoregression uses only one lag of Y in this conditional expectation: E(Yt|Yt-1, Yt-2…)=beta0 + beta1Yt-1; the populatin coefficients can be setimated by OLS
autoregressive distributed lag model
- autoregressive because lagged values of the dependent variable are inclded as regressors, as in an autoregression, and distributed lag because the regression also includes multiple lags of an additional predictor. In genera, an ADL model with p lags of the dependent variable Yt and q lags of an additional predictor Xt is called an ADL(p,q) model
- Yt = beta0 + beta1Yt-1 + beta2Yt-2 + … + betapYt-p + delta1Xt-1 + delta2Xt-2 + … + deltaqXt-q + ut
- the assumption hta the errors in the ADL model have a conditional mea of 0 given all past values of Y and X, that is, that E(ut|Yt-1, Yt-2,…,Xt-1, Xt-2,…)=0 implies taht no additional lags of eiher Y or X belong in the ADL mode. In other words, the lag lenghts p and q are the true lag lengths adn the coefficients on additional lags are 0
least squares assumptions for forecasting with time series data
- Yt = beta0 + beta1Yt-1 + beta2Yt-2 + … + betapYt-p + delta1Xt-1 + delta2Xt-2 + … + deltaqXt-q + ut
- E(ut|Yt-1, Yt-2, …, Xt-1, Xt-2, …)=0
- u has a conditional mean of 0 given the history of all the regressors
- the random variables (Yt, Xt) have a stationary distribution and (Yt, Xt) and (Yt-j, Xt-j) become independent as j gets large
- stationary: so that the distibution of the time series today is the same as its distribution in the past. This assumption is a time series version of the identically distributed part of the i.i.d asssumption: the cross-sectional requirement of each draw being identically distrubiuted is replaced by the time series requirement that the joint distribution of the variables including lags, not change over time. If the time series variables are nonstationary, then one or more problems can arise in time series regression, including biased forecasts
- assumption of stationarity implies taht the conditional mean for the data used to estimate the model is also the conditional mean for the out-of-sample observation of interest. Thus, the assumption of stationarity is also an assumption about external validity and it plays a role in the first least squares assumption for prediction
- 2nd assumption is sometimes referred to as weak dependence, and it ensures that in alrge samples there is sufficient randomness in the data for the law of large numbers and the central limit theorem to hold
- this replaces the cross-sectional reuqirement htat the variables be independently distributed from one observation to the next with the time series requirement that htey be independently distributed when they are separated by long periods of time
- stationary: so that the distibution of the time series today is the same as its distribution in the past. This assumption is a time series version of the identically distributed part of the i.i.d asssumption: the cross-sectional requirement of each draw being identically distrubiuted is replaced by the time series requirement that the joint distribution of the variables including lags, not change over time. If the time series variables are nonstationary, then one or more problems can arise in time series regression, including biased forecasts
- large outliers are unlikely, i.e. Xt,…,Xkt and Yt have nonzero, finite fourth moments
- there is no perfect multicollinearity
estimating the MSFE method 1: by SE of the regression
- focuses only on future uncertainty and ignores uncertainty associated with estimation of the regression coefficients
- attachment
- because the variance of the OLS estimator is proportional to 1/T, the 2nd term in the equation is proportional to 1/T. Consequently, if the number of observations T is large relative to the number of autoregressive lags p, then the contribution of the 2nd term is small relative to the first term. That is, if T is large relative to p, simplifies to the approximation MSFE=sigmau2 This simplification suggests estimating the MSFE by MSFESER = suhat2, where suhat2 = SSR/(T-p-1), where SSR is the sum of squared residuals of the autoregression. The statistic Suhat2 is the square of the SE of the regression (SER)

estimating the MSFE method 2: by the final prediction error

estimating the MSFE method 3: by pseudo out-of-sample forecasting
- uses data to stimulate out-of-sample forecasting. Divide data set into two parts: Initial estimation sample is used to estimate the forecasting model, which is then used to forecast 1st observation in the reserved sample. Next, estimation sample is augmented by 1st observation in reversed sample, and the model is reestimated and is used to forecast the 2nd observation in the reversed sample. This procedure is repeated until the forecast is made of the final observation in the reserved sample and produces P forecasts and thus P forecast errors. Those P forecast errors can then be used to estimate the MSFE. This method of estimating a model on a subsample of the data and then using that model to forecast on a reserved sample is called pseudo out-of-sample forecasting: out of sample because the observations being forecasted were not used for model estimation but pseudo because the reserved data are not truly out-of-sample observations.
- Compared to squared SER estimate from method 1, and final prediction error estimate in method 2, the pseudo out-of-sample estimate in this equation has both advantages and disadvantages.
- advantages: does not rely on the assumption of stationarity, so that the conditional mean might differ between the estimation and the reserved samples. E.g. coefficients of the autoregression need not be the same in the two samples, and the pseudo out-of-sample forecast error need not have mean 0. Thus, any bias in the forecast arising because of a change in coefficients will be captured by MSFEPOOS but not by the other two estimators.
- Disadvantages: more difficult to compute: estimate of MSFE will have greater sampling variability than the other two estimates if Y is, in fact, stationary (because the estimate of MSFEPOOS uses only P forecast errors); requires choosing P. The choice of P entails a trade-off between the precision of the coefficient estimates and the # of observations available for estimating the MSFE. In practice, choosing P to be 10% or 20% of the total number of observations can provide a reasonable balance between these two considerations.

forecast uncertainty and forecast intervals
- In any estimation, it is good practice to report a measure of uncertainty. One measure of uncertainty of a forecast is its RMSFE. Under the additional assumption that the errors ut are normally distributed, the estimates of RMSFE can be used to construct a forecast interval that contains the future value of the variable with a certain probability
- One important difference between forecast interval and CI: usual formula for 95% CI (estimator±1.96 SE) is justified by the central limit theorem and therefore holds for a wide range of distributions of error term. In contrast, because forecast error include the future value of the error, computing a forecast interval requires either estimating the distribution of error term or making some assumption about that distribution.

estimating lag length using information criteria - intro
- how many lags to include in a time series regression? Choosin order p of an autoregression requries balancing MB of including more lags against MC of additional estimation error.
- If the order of an estimated autoregression is too low, you will omit potentially valuable information. If too high, you will be estimating more coefficients than necesary, which in turn introduces additional estimation error into your forecasts
determining the order of an autoregression - F-statistic approach
start with a model with many lags and perform hypothesis tests on the final lag. If not significant, drop it and estimate the next lag. The drawback to this method is that it will tend to produce large models
determining the order of an autoregression - BIC

determining the order of an autoregression - AIC
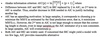
lag length selection in time series regression with multiple predictors
- tradeoff involved lag length choice here similar to that in an autoregression: using too few lags can decrease forecast accuracy because valuable information is lost, but adding lags increases estimation error.
- F-statistic approach: one way to determine the # of lags is to use F-statistic to test join hypotheses that sets of coefficients are 0. In general, th F-statistic method can roduce models that are large and thus have considerable estimation error.
- Information criteria: If the regression model has K coefficients (incl. intercept), BIC(K) = ln(SSR(K)/T) + K(ln(T)/T). The AIC is defined the same way, but with 2 instead of ln(T). The model with the lowest value of BIC is preferred.
- two important considerations when using information criterion to estimate lag lengths
- as in case for autoregression, all candidate models must be estimated over same sample - the number of observations used to estimated the model, T, must be the same for all models
- when ther are multiple predictors, this approach is computationally demading because it requires computing many different models (many combinations of lag parameters). In practice, convenient shortcut is to require all regressors to have the same # of lags, that is to require that p=q1=…=qK so taht only pmax + 1 models need to be compared
- two important considerations when using information criterion to estimate lag lengths
Topics of this course
- Introduction: time series, lags and rst dierences, logarithms and growth rates
- Autocorrelation
- Stationarity
- Dynamic models for forecasting: autoregressive model, autoregressive distributed lag model (ADL), vector autoregressive model (VAR)
- Model selection in dynamic models
- Forecasting
- Dynamic models for analyzing structural relationships
- Interpretation of regression coecients in dynamic models
Total number of observations equals T: usually T << N, but depends on the frequency
why? look up
what can time series regression models be used for?
- estimating dynamic causal effects
- forecasting
OLS assumptions in time series
- OLS assumptions
- E(ui|X1i,X2i,…,Xki) = 0
- (Yi,X1i,X2i,,…,Xki) i.i.d.
- Large outliers are unlikely
- No exact linear relation between X1i, X2i,…, Xki or no perfect multicollinearity
- Questions:
- Are these assumptions realistic in a time series reflecting e.g. a business cycle? Likely to have serially correlated errors since ut depends on ut-1. This causes heteroskedasticity, and violates the i.i.d. assumption. Inconsistent but unbiased.
- What is the consequence of heteroskedasticity?
- What is the consequence of serial correlation?
- What is the relevance of the fourth assumption?
logarithms and growth rates
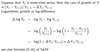
sample autocorrelation

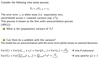
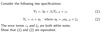




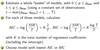


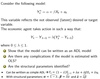
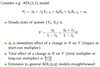



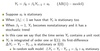


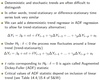
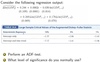
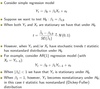
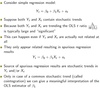


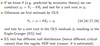

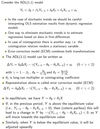
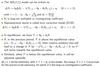

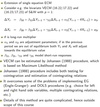