METHODS Flashcards
Method definitions, theories, assumptions, limitations, procedures, tips and tricks, etc.
Demand Characteristics
When the participant changes their true response due to how they believe the experimenter wants it to go.
Expectancy Effects
When the experimenter’s biases cause them (consciously or unconsciously) to sway the experiment to match how they expect it to go.
Regression Discontinuity Design
A type of quasi-experimental design where you create an artificial control group by looking at people who fall close to a particular cutoff score, given that the cutoff score elicits the treatment. (ie, study outcomes of students with a grade of 79% compared to those at 80% who received a scholarship)
What are the 5 types of Quasi-experimental design?
Single group without a control Multigroup without a pretest Multigroup with control and pretest Time series designs Regression discontinuity
What are the 3 ingredients necessary for a randomized experimental design?
Random assignment Manipulates the independent variables Measurement of dependent variables
Grounded Theory of Causal Generalization
Shadish, Cook, and Campbell, 2002 1. Surface Similarity 2. Ruling Out Irrelevancies 3. Making Discriminations 4. Interpolation & Extrapolation 5. Causal Explanation
Multicollinearity
When there is a very high (or even perfect) correlation between independent variables. It’s a good indication that the data is not reliable. (Edwards 2008)
Construct Validity
the degree of correspondence between the constructs (i.e., latent traits) referenced by a researcher and their empirical realization (i.e., operationalization/measure); in other words, is it measuring what it claims to be measuring?(Stone-Romero, 2011)
Bias
systematic variance in an operational deginnition that is unrelated to the focal construct (Stone-Romero, 2011)
Unreliability
nonsystematic (random) variance in an operational definition (Stone-Romero, 2011)
Deficienct
when the constructs empirical realization (operationalization/measure) does not fully capture the essence of the construct (Stone-Romero, 2011)
Special Purpose vs. Non-Special Purpose Settings
More appropriate than a field vs lab distinction SP- created for the specific purpose of doing research (e.g., university labs, simulated work settings in an org); designed to allow for the effective (unconfounded) manipulation of one or more independent variables NSP- created for the purposes other than reserach (e.g., organizations, classrooms, churches, etc. )
Probability sampling
workers are selected from a population (with N members) in a way that ensures that the probability of selection a sample of a given sze (e.g., n=10) is equal to the probbilty of selection any other sample of the same size… I.E., all people in the population have an equal chance at being selected to participate– rarely occurs in IO for many obvious reasons
Startified random sampling
a researcher specifies strate of employees within an org (e.g., managerial, nonmanagerial) and then randomly select a specific number of workers from each stratum of interest
multistage cluster sampling
a researcher a) selects a random sample of units in one or moer clusters (e.g., private vs. public-sector, profit vs. non profit) and then b) randomly selects clusters of orgs from each larger cluster
nonprobabilty sampling
uses non random strategies for selecting sample members from a target population e.g., convenience sampling, purposive sampling of heterogeneous instances, systematic sampling, quota sampling
convenience sampling
selecting a nonrandom sample based on their availability to to participate in a study (i.e., intro to psych students)
purposive sampling of heterogeneous instances
selecting a nonrandom sample of members on the basis that the researcher believes that they are diverse in terms of characteristics that might influence a causal relation between variables (Shadish et al., 2002)
systematic sampling
select sample members in a methodical way from lists of assumed population members (e.g., every 10th person in the SIOP directory)
quota sampling
selecting specific numbers of sample members of different types to produce a sample that is roughly representative of the target population
Critiques of IO rigorous reserach
a) has little to no practical utility b) does not inspire confidence in the work of scientiests c) distorts important aspects of reality in orgs d) fails to consider phenomenon that are not readily measurable e) has low releance to the criteria that are important to practitioners f) doesn’t offer much more than common sense/common knowledge g) it is artificial/ irreleant/trivial j) has low operational validity (aka variables that can’t be controlled by practitioners realistically or ethically k) focuses on “proving” the truth of hypotheses and not problem solving l) untimely- deals with issues that change more rapidly than the knowledge it produces m) involves participants who are not representative of the populations to which generalizations are to be made (Stone-Romero, 2011; Thomas & Tymon, 1982)
Critique of NSP Causal Claims
- harder to control extraneous and confounding variables even using random experiments or quasi-experiments - the claim that there is more mundane realism and thus more external validity doesn’t always hold up - typically involce nonrepresentative samples of subjects, settings, and operational definitions of manipulations/measures - Locke (1986) showed evidence that typically relations found in SP settins hold up in NSP settings.
Mundane Realism
Is it consistent with the every day realities (e.g., adding health benefits, vacation time, etc. to simulated orgs even if it is unrelated to the study); if a study lacks mundance realism, an effect found in SP settings may not generalize to NSP settings (Stone-Romero, 2011)
Experimental Realism
the manipulations/measures are operationally defined such that they have a suffidient impact on study participants, it is noticable, it is legitimate enough to show an effect; if it lacks this, models may fail to show support for causal connections between variables, even if there is one i.e., why manipulation checks are important (Stone-Romero, 2011)
3 Major Attributes of Randomized Experimental Designs
- Manipulation of IVs (gives confidence about temporal precedence- causes preceding effects 2. Random assignment of units (individuals, teams, orgs) to study conditions (gives confidence that results are not due to confounds; that conditions are equivalent) 3. Measurement of DVs (outcome measures must be reliable or effects may not be found)
Limitations of Randomized Experimental Designs
- You just can’t manipulate some variables (e.g., sex) 2. Some variables that can be manipuated, shouldn’t be on ethical grounds (e.g., mental health) 3. in NSP settings, may not be able to randomly assign units 4. in NSP it may not be possible to isolate units (spatially), so you can’t be positive about treatment effects 5. participants may refuse to be assigned to experimental conditions on a random basis 6. it may be unethical to withold beneficial treatments from units (i.e., control group receiving no training/treatment they should really get)
Threats to experimental design internal validity
Differential attrition across conditions, history, testing, resentful remodalization, and the interaction of two or more threats (Stone-Romero, 2011)
3 Attributes of Quasi-Experimental Designs
- Manipulation of IVs 2. Nonrandom assignment of units to conditions (why it doesn’t hold as much power to make causal inferences) 3. Measurement of assumed DVs ( assumed because the units are not randomly assigned to we can’t be sure the outcomes are caused by the IV, only assume so)
Nonexperimental Designs
quantitative & qualitative research
Correlational study
studies that consider relations between (among) variables shouldn’t be referred to as correlational studies because corrrelation is a statisical technique; not an experimental design
Nonexperimental Design Attributes
- Measurement of assumed IVs (not manipulating makes them be assumed &hard to prove temporal precedence) 2. Nonrandom assignment of units to assumed conditions (collecting data from participants with an assumed level of the IV that could have stemmed from various causes) 3. Measurement of assumed DVs (not randomly assigned so we can’t be sure the DVs are caused by the IVs)
Limitations of Nonexperimental Designs
You really can’t make causal claims/inferences because of the lack of internal validity and the inabilty to prove temporal precedence, control confounds (only able to confidently demonstrate correlation/covariation)
Design vs. Method
Research Design and Statistical Methods are independent; Studies of each type of design can use a variety of statistical methods to analyze the data.
Prediction vs. Causal Claims
Prediction is a statistical term meaning inforation on a set of predictor variables can be used to perdict the value of a ctierion of interest This does not equate to evidence of a cause and effect relationship.
Causal claims
Need to be able to prove temporal precedence, covariation, and rule out any alternative explanations (control for confounds) Internal validity is driven by experimental design and cannot be compensated with external validity. The use of causal models (e.g., regression, MLM, SEM) does not justify the use of causal claims/inferences
Most frequent IO designs
Nonexperimental studies (esp. quantitative studies) are most common and NSP (field) settings being most common (Stone-Romero, 2011) Quasi-Experimental designs seem to be the least common
“Ranking” of Validity
If a study does not have internal, construct, and statistical conclusion validity; the external validity is irrelevant.
4 types of Validity
Internal validity ( relating to the existense of cause-effect relationships between variables) Construct validity (correspondence of constructs with operationalizations) Statistical Conclusion validity (the correctness/confidence/trueth of statistical estimates derived from the study) External validity (the extent to which relations generalize across different settings, populations) (Stone-Romero, 2011)
Stone-Romero (2011) Suggestions for Minimizing Common Method Variance
1) obtain predictor and criterion data from different sources 2) separate the time at which predictor and criterion variables are measured 3) counterbalance the order in which variables are measured
Threats to Statistical Conclusion Validity
a) low statistical power (i.e., small sample sizes) b) failing to mett the assumptions of the stats test c) conducting a large # of stats tests using a Type I error rate tha is lower than the actual (effective) Type I error rate
Statistical significance & practical importance
They are not mutually excluse There are “non-sig” effects that are practically important There are sig-effects that have effect size so small they make them not practically important See (Wasserstein et al., 2019) for more on why p-values/statistical significance is problematic.
Why is MTMM problematic?
1) Assumes traits and methods are uncorrelated 2) No set “rules” for what is enough in terms of satisfying rules (Stone-Romero, 2011)
Detecting Method Variance
1) MTMM 2) CFA
4 Attributes of Qualitative Research
- Occurs in natural settings (most of the time) 2. data is derived from the participants perspective 3. should be reflexible (i.e., flexible, dynamic) 4. qualitative instruments, observation methods, and modes of analysis are not standardized; the researcher serves as the main research instrument
Typical Purpose of Qualitative Research
Theory generation or Theory elaboration; not as suitable for theory testing (Lee et al., 2011)
3 most common qualitative designs in IO
Case Studies Ethnographies (observations) in-depth interviews
Grounded Theory approach to Qualitative Research
on a regular, iterative basis it requires a) hypothesis generation from data b) testing hypotheses on new data that may elicit revisions in OG hypotheses c) testing revised hypotheses with new data continue this until there’s theoretical saturation or no new learnings occur (Lee et al., 2011)
Qualitiatve vs. Quantitative questions
- What is occuring? How is it occuring? What constructs should I use to explain it? 2. What is the prevalence of this? Does this generalize?
Qualitative limitations
- Very time intensive 2. Can be professionally risky (takes away from other research methods, takes so long, harder to publish) 3. some org scholars claim we have too much theory, but it is possible to test theory through qualitative means as well (Lee et al., 2011)
MTMM Rule for Showing Convergent Validity
Validity Diagonal must not = 0 and they should be sufficiently large (Campbell & Friske, 1959)
MTMM Rules for Discriminant Validity
- The validity diagonal > adjacent rows/columns in the hetero-trait/hetero-method triangles 2. The validity diagonal> corresponding heterotrait/monomethod triangles 3. the same pattern of trait interrelationships should be shown in all heterotrait triangles in both the monomethod blocks and heteromethod blocks (Campbell & Friske, 1959)
Calculating Method Variance with MTMM
heterotrait monomethod values- heterotrait heteromethod values = method variance (Campbell & Friske, 1959)
MTMM Tips and Tricks
- You can view heterotrait monomethod triangles as method factors– if its the highest correlation, it means method is mostly driving it
- the smaller the values in the heterotrait/heteromethod triangles, the more indepedence there is (whether it is the traits or the methods)
- if heterotrait/hetero method triangles are close to equivalent with validity diagonal that implies there is no discriminant validity
- if heterotrait/ monomethod traingles are about the same or close to equivalent to reliability values, that implies there is no discriminant validity
(Campbell & Friske, 1959)
MTMM logic summary
Measures of the same trait should correlate higher with each other than measures of different traits with different methods Ideally, the validity values should also be higher than the correlations among different traits measured by the same method ** these conditions are rarely met and therefore it is a subjective judgement in the end about what is “valid enough” (Campbell & Friske, 1959)
MTMM Assumptions
- Traits and Methods are independent/uncorrelated 2. You are interpreting validites with the reliabilty of methods in mind 3. Unreliable methods makes the whole procedure a bit pointless it is concerned with the adequacy of tests as measures of a construct, rather than the adequacy of a construct (Campbell & Friske, 1959)
Reliability and Validity in relation to agreement
Reliability is agreement between two efforts to measure the same trait through maximally simlar methods Validity is represented in the agreement between two attempts to measure the same trait through maximally different methods (Campbell & Friske, 1959)
Topics/Trends of 1917-1925
- Objectivity and methods to solve important problems
- Cognitive ability tests
- Interest in the exceptional
- beginning of statistical significane and prediction models
- sources of rating errors (e.g., halo (Thurston, 1920) and guessing)
- focus on psychometric properties, classification, and test equivalence (Cortina et al., 2017)
Topics/Trends of 1925-1945 (The Depression and WWII)
- Test scoring methods, test form equivalence, and cross-validation
- assessment of non-cognitive testing (e.g., social/emotional intelligence, values, interests)
- limitations of self report measures
- Shift to a hypothetico-deductive method (i.e., hypothesis testing and theory driven studies)
- properties of distributions (e.g., growth curves, Poisson)
- Reflections on the past: too much survey work, need to expand samples of interest (Cortina et al. 2017)
Topics/Trends 1946-1969
- Predicive power of personality measures (esp. MMPI)
- Social desirability and faking
- development of many new measures (OPQ, PAQ)
- literature reviews become common
- science of job attitudes
- statistical significance over effect size (lol a start of the issue)
- smaller font for method section (showing the shift to focus of theory at the expense of methods)
- Reflections on the past: we stopped studying the exceptional, decline in practitioner authorship, shortened time perspective for the field (Cortina et al. 2017)
Topic Trends 1970-1989
- Focus on measure development (job characteristics, work values, job involvement)
- IRT
- self vs. supervisor reports,
- # of points and anchors in a rating scale
- increased focus on methods-specific papers
- data-analytic innovations of meta-analysis and SEM (Cortina et al. 2017)
Topic/Trends of 1990-2014
- Methodological plurality (no single approach no matter its potency is the right answer for all questions/research)
- Broadening of methodological choices
- -introduction of methods-oriented journals -
- publication of reactive and prescriptive reviews of methodological practices
- realization that data analytic approaches do not occer a pancea to solve research issues -
- subgroup differences -
- level of analysis, aggregation, and MLM -
- importance of effect sizes
- restoration of method section font size (Cortina et al. 2017)
Suggestions for 2017 and Beyond
- Replication of research -understanding the exceptional, both good and bad -identifying inappropriate methods in the review process (method experts review each paper; pre-review before starting) - better research design and measurement - more specific theories that are testable rather than offerly vague ones that could never by fully validated (Cortina et al. 2017)
Critique of Maximum Likelihood Estimation for Validity
- Only uses sample derived information in making inferences/decisions about test validity; ignoring all relevant info accumulated in the past (Schmidt & Hunter, 1977)
Bayesian Approach for Validity Generalization
- utilizes sample data and prior knowledge in estimating validity & weighs each in proportion to its information value - prior distribution of variances is combined with sample info to create a posterior distribution - the mean (or mode) of the posterior distribution is taken as the estiate of the parameter of interest (i.e., test validity) (Hunter & Schmidt, 1977)
Why is Bayesian for Validity a good idea?
- bayesian priors used are based on data (i.e. not subjective) and incorporates all past relevant info
- the assumptiosn made about between study variance in criterion reliability and range restriction are conservative
- certain sources of error variance in the obtained distribution are not corrected for, making it even more conservative
- corrections made to the mean of the prior for average range restriction effects are “probably” conservative
- procedures provides a parsimonious, sophisticaed and tech sound solution for the overarching problem of validity generalization
- the model can be extended to provide an improved method of data analysis and decision making in criterion-related validity studies
- when slightly modiffied, it provides a tool that can lead to the establsihment of general principles about trait-performance relationships (Hunter & Schmidt, 1977)
Correcting for error variance due to sample size
Find the average sample size across published studies then take 1(N-3) to get the estimates variance due to the sample size (Hunter & Schmidt, 1977)
Notion for correcting for error variance (i.e., reliabilty and range restriction) in validity generalization
If you take a bunch of validity coefficients, standardized them into Z scores, and then subtract the error variance and the variance of the distribution of the validiy coefficient is 0- then essentially it shows there is no true variation in validation; therefore, if we correct these in the beginning we are more likely to find the true validity ((Hunter & Schmidt, 1977)
Correction Warning
corrected variance tends to overestimate rather than underestimate (Hunter & Schmidt, 1977)
Common Method Variance
variance that is attributed to the measurement model rather than to the constructs the measures represent (Podsakoff et al., 2003)
Effects of Common Method Variance
Method effects inflate the observed relationship when the correlation between the methods is higher than the observed correlation between methods -method effects; it deflates the observed relationships when the method correlations are lower than the observed correlation- method effects (Podsakoff et al., 2003)
Common method biases causes
common rater common measurement context common item context characteristics of the items themselves (Podsakoff et al., 2003)
Stages of the Response Process
Comprehension Retrieval Judgement Response Selection Response Reporting (Podsakoff et al., 2003)
Comprehension Stage Potential Method Bias
Item ambiguity
Retrieval Stage Potential Method Bias
measurement context, question context, item embeddedness, item intermixing, scale size, priming effects, transient mood states, and item social desirability
Judgement state potential method biases
consistency motif ( in attempt to increase accuracy in the face of uncertainty), implicit theories, priming effects, item demand characteristics, and item context-induced mood states
Response selection potential method biases
common scale anchors and formats; item context-induced anchoring effects
Response reporting potential method biases
consistency motif (in an attempt to appear rational), leniancy bias, acquiescence bias, demand characteristics, and social desirability
Two main ways to control for method biases
a) Study design b) statistical controls never rely on one or the other; a combo of both is best
Common Rater Effects
Consistency Motif Implicit theories (& illusory correlations) social desirability leniency bias acquiescence biases (yea-saying and nay-saying) mood states (i.e., PANAS) transient mood states
Item Characteristic Effects
Item social desirability item demand characteristics item ambiquity common scale formats common scale anchors positive and negative item wording
Item Context Effects
- Item priming effects
- item embeddedness
- context-induced moods
- scale length (shorter is better for fatigue/boredom, but may enhance the likelihood of previous items influencing responses)
- Intermixing (or grouping) of items or constructs on the questionnaire
Measurement Context Effects
Predictor and criterion variables measured at the same point in time predictor and criterion variables measured in the same location predictor and crierion variables measured using the same medium
Crique of Harman’s Single Factor Test
The use of EFA/CFA to see if the most variance loads on one factor is problematic because it assumes if there is method variance it would account for most/all the covariance which is a large and inappropriate assumption to make; it doesn’t control for the method variance; just identifies it * yet it is still one of the most widely used methods for detecting method bias (Podsakoff et al., 2003)
Partial Correlation Procedures for Method Bias
Partialling out social desirability as a surrogate of method variance Partialling out an unrelated “marker” variable that is unrelated to the construct of interest Partialling out a general methods factor compares the structural parameters with and without these factors to determine potential effects (Podsakoff et al., 2003)
Controlling for a measured latent methods factor
items are allowed to load on their theoretical constructs and a latent method factor (with its own measurement component) and the sigficance of the structural parameters are examined with and without the latent factor; the latent factor is assessed by a surrogate variable (e.g., social desirability) assumed to represent CMV (Podsakoff et al., 2003)
Controlling for an unmeasured latent methods factor
Items are allowed to load on their theoretical constructs and a latent common methods variance factor’ structural parameters are compared with and without the methods factor’ the variance of the responses is partitioned into trait, method, and random error (Podsakoff et al., 2003)
CFA x MTMM
Combines both CFA and MTMM to partiion variance into trait, method, and random permitting researchers to control both for method variance and random error when examining relationships between predictor and criterion variables (Podsakoff et al., 2003)
Correlated Uniqueness Model
Each observed variable is caused byonle one trait factor and a measurement error term; there are no methods factors; the model accounts for method effects by allowing the error terms of constructs measured by the same method to be correlated (Podsakoff et al., 2003)
Direct Product Model
this model assumes the trait measures interact multiplicativel with the methods of measurement to influence each observed variable. The model assumes stronger the correlation between the traits, the more intercorrelation between the traits will be infuenced by shared method biases (trait and method components can’t be separated in analysis can’t control for method factors while testing for relationships; does not test for interactions after first controlling for main effects) (Podsakoff et al., 2003)
Partial Correlation Approaches Disadvantages
fails to distinguish between method bias at the measurement level from method bias at the construct level ignored measurement error in the method factor only control for single source of method bias at a time ignores method x trait interaction - besides Harmon’s single factor solution, these solutions are the worst (Podsakoff et al., 2003)
Single Method Scale Score Approaches disadvantages
-ignores measurement error in the method factor - only controls for a single source of method bias at a time - ignores method x trait interaction (Podsakoff et al., 2003)
Single method factor approaches disadvantages
- only controls for a single source of method bias at a time - ignores method and trait interactions (Podsakoff et al., 2003)
Multiple-method factor approaches disadvantages
Ignores method x trait interactions (i.e., your best bet because these approaches leverage a MTMM approach in a way) (Podsakoff et al., 2003)
4 Questions to ask when determining the right CMV remedy
- Can the predictor and criterion variables be obtained from different sources? 2. Can the predictor and criterion variables be measured in different contexts? 3. Can the sources of method bias be identified? 4. can the method bias(es) be validly measured?
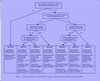
Mediator
an intervening variable that transmits the effects of an antecedent variable on to a dependent variable X->M->Y
Moderator
a variable that causes the relationship between X and Y to vary; whether is it the direction or the magnitude (also a statistical interaction)
A moderated mediation
when the magnitude or direction of the mediation effect is dependent on the values or level of a moderator (e.g., motivation -> job performance-> salary, but gender moderates job performance such that the job performance-> salary relationship is weaker for women than men) ** completely made up not based on a study
A mediated moderation
a mediated interaction; basically when the X x Z interaction is causing the M (mediator) which is then causing the Y e.g., SJT test format x race -> face validity (reading comprehension -> test performance (Chan & Schmitt, 1997)
Alpha Change
changes in absolute levels given a constant conceptual domain and a constant measuring instrument (Golembiewski et al., 1976; Chan, 2011)
Beta Change
changes in absolute levels that are driven by an alteration of the respondents scaling; a mental recalibration of the scale (Golembiewski et al., 1976; Chan, 2011)
Gamma Change
changes in the conceptual domain (i.e., changes in the meaning or conceptualization of what the construct is ((Golembiewski et al., 1976; Chan, 2011)
Baron & Kenny (1986) Mediation Test
- use regression/correlation to test for the seperate significant relationships between a) X and Y b) X and M c) M and Y controlled for X 2. if one or more of the above relationships is non-significant–> no mediation 3. if significant; one should test the significance of a) M and Y controlling for X b) X and Y controlling for M 4. if a) is significant and b) is non-significant–> mediation!
Baron & Kenny Mediation criticisms
- very low ability to detect mediated effects 2. does not provide a numerical esaimte of the strength of the meditation (i.e., indirect effect) 3. requires the overall X and Y is significant, however, technically a causal chain between X ->M->Y where X –> Y don’t have a significant relationship could surely exist OR it could exist at the population level but the sample level doesn’t detect it because of small effect size/low power
OLS Regression Assumptions
- errors are assumed to be normally distributed with a mean of 0 (normality assumption) 2. error variances are constant across different values of X (homoscedasticity assumption) 3. the errors from each observation are not correlated with the errors from the other sample observations (i.e., independence assumption violating the independence assumption is the most impactful violation you can make (Chan, 2011)
What types of methods/tests are used for testing Interactions?
When X and Z are categorical -> ANOVA When either X or Z are continuous -> multiple regression or hierarchical multiple regression
Proving causal claims in quasi-experimental designs
Designs without control groups can yield strong causal inferences ONLY by reducing the plausibility of alternative explanations for the treatment effect. ○ To reduce the plausability of alternative explanations in quasi-experiments is: - Identification and study of plausible threats to internal validity - Primacy of control of design □ e.g., adding elements such as observation at more pretest time points, additional control groups - Coherent pattern matching □ A complex prediction is made about a given causal hypothesis that few alternative explanations can match (e.g., nonequivalent dependent variables) (Shadish et al., 2002)
Ιdentify the relevant components in the ΜΤΜΜ matrix
draw conclusions on the validity

Τhere is convergent validity
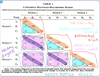
Identify the relevant MTMM components and make appropriate validity inferences


Time Series Design
- A large series of observations made on the same variables consecutively over time
- Key is knowing the specific point in the series at which a treatment occurred.
If the treatment had an effect, the causal hypothesis is that the observations after treatment will have a different slope or level from those prior to treatment;
A series should show an interruption to the prior situation at the time at which treatment was delivered
Time Series Design Effects
Types of effects:
- Should be a sharp discontinuity at the point of the intervention (called a change in
level or intercept)
- Should be a change in slopes of the series at the point of interruption (called a drift,
trend, or slope)
- Continuous effect does not decay over time
- Discontinuous effect does not persist over time (common if intervention is
introduced and then removed)
- Immediate or delayed (more difficult to interpret) effects -
Form
Describe the effect on all three of the above dimensions
of the effect (level, slope), its permanence (continuous vs discontinuous) and its immediacy
(immediate or delayed)
We must design studies that MAXMINCON
(Shadish et al. 2002)
1) MAXimize systematic variance
i. Collect information on variables that may affect the treatment
ii. Variance you can account for is systematic; variance unaccounted for becomes error
2) MINimize error variance
i. Use highly reliable measures
ii. Use multiple measures of the same construct
3) CONtrol extraneous variance
i. Control any influences that many impact our outcome
Surface similarity
i. Scientists generalize by judging the apparent similarities between what’s being studied and the target of the generalization
1) E.g., the effects of secondhand smoke in the workplace seems more similar to public settings at issue in policy debates than do studies of smoking in private residences
Ruling out irrelevancies
(shadish et al., 2002)
Identifying those attributes of persons, settings, treatments, and outcomes that are irrelevant because they do not change a generalization
E.g., Size is irrelevant to the membership of the bird category
Making discriminations
Shadish et al 2002
Scientists generalize by making discrimination that limit generalization. 1) Child psychotherapy works in a lab but might not work in the clinic
Interpolation and extrapolation
Scientists generalize by interpolating to unsampled values within the range of the sampled persons, settings, treatments, and outcomes, and much more difficult, by extrapolating beyond the sampled range
1) The effects of therapies for cancer increase as the dose increases until the point at which they would cause the patient to die (interpolation) or the effects of toxic chemicals on small animals will generalize to humans (extrapolation)
Causal explanations
Scientists generalize by developing and testing explanatory theories about the.target of generalization
1) A theory applies to both scratching in primates and rearing in rats because the biological mechanisms underlying both these behaviors is the same.
Shadish et al 2002 take on validity
- “The approximate truth of an inference”
- When we say something is valid, we make a judgment about the extent to which relevant evidence supports that inference as being true or correct
- It is a property of inferences, not a property of design or methods
Statistical conclusion validity
- “The validity of inferences about the correlation (covariation) between treatment and outcome”
- Refers to the appropriate use of statistics to infer whether the presumed independent and dependent variables covary
Construct validity
shadish et al. 2002
the validity of inferences about the higher order constructs that represent sampling particulars
Internal validity
shadish et al., 2002
○ The validity of inference about whether or not the covariation resulted from a causal relationship
External validity
The validity of inferences about whether the cause-effect relationship holds over variation in person, places, setting, treatments, and measurement variables.
Three major aspects of construct validation
Nunnally, 1978
○ Specifying the domain of the construct
○ Empirically determining the extent to which items measure that domain
○ Examining the extent to which measure produce results that are predictable from theoretical measures
Factor Analysis vs. Principle Components Analysis
Differences in analysis
1) The differences lie in the variance that is analyzed
PCA:
□ ALL the variance in the observed variables is analyzed…which is why there is 1.0 in the diagonal of the correlation matrix– assumes each item is perfectly correlated with itself
FA:
□ Only SHARED variance is analyzed
□ Uses an estimate of the variance in each items shared with other items as the diagonal of the correlation matrix
Called a ‘communality estimate’
Factor analysis vs. Principle Component Analysis
Theory
Tabachnick & Fidell, 2001
Theoretical differences in the reason that the variables are associated with a factor or component
- *a. FA: reflexive structure**
a) Factors cause the variables - *b. PCA: formative structure**
a) Variables produce the component
There is NO underlying theory about which variables should be associated with which factors (more about data reduction and categorization not identifying underlying latent traits)
EFA vs CFA
EFA
Theory Development purposes
□ One seeks to describe and summarize data by grouping together variables that are correlated (used in early stages of research)
○ Confirmatory
Theory testing purposes
□ Used to tests a theory about latent processes □ Often performed through SEM
Eigenvalue
an index of the strength of the factor; Put differently, the amount of variance for which the factor accounts
- we retain factors with eigenvalue greater than 1
Rotations in Factor Analysis
process by which the solution is made more interpretable without changing underlying mathematical properties
- Orthogonal: factors are uncorrelated
- Varimax most common
- Oblique: factors are correlated
- Promax rotation most common
Determining the number of factors in EFA
Hinkin (1998)
1) Kaiser criterion: keep factors with eigenvalues greater than 1
- This criterion isn’t always useful
- Some authors have found that Kaiser’s approach can lead to over- factoring
- It is based on assumptions pertaining to PCA
2) Scree test: look for the dip.
3) Also, percent of total and common variance accounted for by the factor
4) And interpretability (are all factors retained interpretable?)
What items to retain in scale development with EFA
Hinkin 1998
1) Factor Loadings: only items that clearly load on a single factor (grater than factor loading of .40 and/or loading twice as strong on the appropriate factor than any other factor)
- a. .4 or .45 are common cutoffs
- b. More than .5 is really good
- c. Large and positive loadings for the “correct” measures
2) Communality stats: this helps determine the proportion of variance in the variable explained by each of the items; retain items with higher communalities
3) Percentage of total item variance: larger the better ( > 60% is good)
Effects sizes are linked to
- The sample size on which the effect size is based
- Alpha- level: The probability at which we accept an effect as being
- Statistical power: The ability of a test to detect and effect of that size
Item generation approaches
Hinkin 1998
2 approaches to generate preliminary items
1) Deductive
a) Review literature and theory to acquire a full understanding of the construct (e.g., finding the definition of expert power) and find further examples of behaviors that fit the definition
2) Inductive
a) Simply ask people for examples of behavior
i) Useful when conceptual basis of construct may not be easily identifiable and exploratory research
ii) E.g., asking respondents to describe “how your manager
communications with you”
Item development recommendations
Hinkin 1998
1) Simple, short statements
2) Familiar language
3) Avoid double-barreled items
Number of items for scales
Hinkin 1998
1) Short measures are effective at minimizing response bias caused by fatigue and boredom
2) At least four items are needed to test the homogeneity of items within a latent construct (by item intercorrelations) (Billings & Nilan, 1985)
a) Scales can have high internal consistency even with low item intercorrelations
3) Final goal will be to retain about four to six items for most constructs, but the final determination must be made only with accumulated evidence to support construct validity (Schriesheim et al., 1993)
4) Always generate twice as many items as you may need
Scale development - internal consistency assessment
Hinkin 1998
i. Reliability: the accuracy of precision of a measuring instrument and is a necessary condition [but not sufficient] for [construct] validity
1) Establish reliability after unidimensionality has been established
ii. Can calculate a number of ways but internal consistency using Cronbach’s alpha is the most commonly accepted measure (Price & Mueller, 1986)
1) A large coefficient alpha (minimum of .70 for exploratory measures; Nunnally, 1978) provides and indication of strong item covariance and suggests that the sampling domain has been captured adequately (Churchill, 1979)
2) May want to eliminate items that reduce reliability, especially if these additional items lower interitem correlations
3) Alpha is very sensitive to the number of items (Cortina, 1993)
EFA vs PCA
PCA is more for data reduction, minimizes the values, the goal is to produce a set of components that can be used to reproduce the data matrix
EFA- derive a set or factors that can be used to produce the covariance matric; infer what we can’t see from what we can see; identify the underlying commonalities driving the factors
Path Analysis vs SEM
Path analysis- no measurement errors included
SEM- the measurement error is included
Key Difference between Moderation and MLM
- It’s like a MANOVA and multiple ANOVAs
- at a practical concrete level is the error rating and having more statisical power
Rwg
(James et al., 1984)
- The extent to which the group agreed
- the ratior of variance of the group to variance of complete disagreement
- i.e., the ratio of observed disagreement to possible total disagreement
- possible disagreement usually modeled as a null distribution (unified distribution) but at times it is a skewed distribution and not rectangular
- arbitrary rule of .7 or greater to justify aggregating
Interrater agreement vs. Interrater reliabiltiy
Agreement: the extent to which the members of the group giver interchangable responses
- about the absolute value
- shown in terms of distance apart on graphs
- this is what is needed to justify agreement/groups/aggregation
Reliability: the extent to which the group ratings are orrelated with each other
- about the ranking of ratings
- shown in terms of shape on graphs
- aggregation based on this can get you aggregation bias because it can be very high with no interrater agreement present
Intraclass Correlations
ICC(1) vs. ICC (2)
ICC (1)- the variance within groups compared to the variance between groups
- the lower the number is the less variance there is in between groups than within groups; which shows agreement
- proven to be wrong for justifying aggregation- Rwg is a better option
ICC (2) - the reliabilty of the group means or the amount of error in the means
- you want this one to be larger
Parceling in SEM
when you have more than 4-7 items loading on a factor, it becomes impossible to get a good fit
- you take the first three average them = parcel, and so on
- your indicators are then little composites of items (parcels) not items
Ways to replace missing data
- Mean substitution ( worst option)
- imputation
- the process of predicting what the person would have said to that item if they answered based on their responses to all the items they did answer
- E.M. Algorithm ( Expectations maximization)
- considers all possible configurations of values in all the empty slots and comes out with the configuration that best optimizes some sort of criterion
Spector (2010) CWB research
Basically showed common method variance is essentially a myth and doesn’t need to be made into as big of a deal
- conclused we should use frequency ratings, not agreement
- should use self-report not supervisor
- don’t use antithetical items
Reflective indicators (Podsakoff, 2003)
(a) the indicators were conceived of as manifestations of the construct, (b) changes in the indicator
were not expected to cause changes in the construct, (c) changes in the construct were expected to cause changes in the indicators, (d) the indicators shared a common theme, (e) eliminating an indicator was not expected to alter the conceptual domain of the construct, (f) a change in the value of one of the indicators was expected to be associated with a
corresponding change in all of the other indicators, and (g) the indicators were expected to have the same antecedents and consequences.
Formative indicators
Posdakoff 2003
(a) the indicators were viewed as defining characteristics of the construct
(b) changes in the indicators were expected to cause changes in the construct,
(c) changes in the construct were not expected to cause changes in the indicators,
(d) the indicators did not necessarily share a common theme,
(e) eliminating an indicator might alter the conceptual
domain of the construct,
(f) a change in the value of one of the indicators was not necessarily expected to be associated with a change in all of the other indicators,
(g) the indicators were not expected to have the same antecedents and consequences.
CTT Limitations
1) Item and person statistics are interdependent
- a. Can’t compare items from different samples
- b. Can’t compare persons who take different tests
2) Reliability is flawed and you always get lower-bound estimates
3) Standard error of measurement same for all persons
- (despite how well that persons ability matches up with item difficulty)
- Is based on reliability which is a test property that doesn’t consider the person or persons in question
4) Is test oriented, not item oriented
* a.Goal is to design test. We know nothing about probability of responses to individual items
5) If test scores are not equally precise across individuals (which they are not, given that
items contain more information when matched with the respondents ability), how can we
compare scores across persons within a sample?
Why use Cohen’s D?
- Used as a counterpoint to significance tests where this effect size gives an indication of how big or small a significant difference is.
- This difference can then be compared to Cohen’s (1988) estimates of what is typical of a small (e.g., .2), medium (e.g., .5), or large (e.g., .8) effect. Typically share the same range as standard deviation (-3.0 to 3.0)
- To provide a common metric on which to compare effects for meta-analysis or what not when outcome variables may be measured on different scales.
SEM
(covariance structure analysis)
A hypothesized causal model that specifies directional paths linking all of the study variables is posited based on theoretical arguments by the researcher and fitted to the
observed data.
MultiLevel Modeling (MLM; HLM)
- Evaluates relationships at multiple levels of analysis
- Models variances among variables at these different levels
- Well suited to tests cross-level interactions
- Allows researchers to partition the variance in outcome variables
- Provides method for analyzing longitudinal relationships
When to use MLM?
Why is Ordinary Least Squares (OLS) Regression not appropriate for nested/multilevel data?
1) Errors in OLS are assumed to be independent
* In multilevel data, independence assumption is almost always violated
2) size for determining statistical significance of b2 is the lower level observations which is incorrect, instead of using the group-level sample size
* Using the wrong (inflated) sample size, the standard errors are wrong and it is easier to obtain statistical significance for the effect of z (increasing type 1 error)
Why/When to use MLM
- Observations nested within a larger grouping
- Occasions within ppl (repeated measures), Kids within schools, employees within companies, etc
- Need to account for non-independence of observations (i.e., not assigned at random)
- Balanced data are not required (i.e., groups don’t have to be equal in size)
- Analyses complete data, not just complete data
- Lots of missing data with repeated measures and longitudinal data; takes that into account
Hinkin 1998 on MTMM
Original MTMM guidelines criticized for use of unrealistic assumptions and reliance on a qualitative assessment of comparisons of correlations.
An SEM model is typically composed of what two parts?
- A measurement model – describes how latent variables are measured by their manifest indicators (i.e., test scores, survey observation)
- ·A structural model – describes the relationship between the latent variables and shows the amount of unaccounted for variance.
Measurement Concerns/Challenges
- The Criterion Problem
- Violating Assumptions
- Multicollinearity
- Common Method Variance
- Common Method Bias
- Measurement Invariance
- Inconsistencies in studies/literature
- typically indicates a missing moderator
When should we dichotomize?
(Cohen, 1983)
- to Eases data analysis,
- variable in question is a crude measure
- dichotomy represents true state of affairs
Reverse Coded Items
Spector et al. 1997
- Factor analyses of scales that contain items written in opposite directions sometimes show two factors, each of which contains items written in only one direction.
Schmitt 1996
Issues with how people use alpha
- Alpha is not an appropriate index of unidimensionality to assess homogeneity; it assess internal consistency– those are different
- In correcting for attenuation due to unreliability, use of alpha as an estimate of reliability is based on the notion that the measures involved are unidimensional.
- if it isn’t unidimensional it will lead to overcorrection
- There is no sacred level of acceptable or unacceptable level of alpha. In some cases, measures with low levels of alpha may still be quite useful.
- Presenting only alpha when discussing the relationships of multiple measures is not sufficient. Intercorrelations and corrected intercorrelations must be presented as well
Nunally 1984
- Says reliability should be a minimum or .70 for research
- Should be at least .90 and preferably .95 for making important decisions about individuals.
The Various Descriptions of Alpha (Cortina, 1993)
- Alpha is the mean of all split-half reliabilities
- Alpha is the lower bound of reliability of a test
Internal consistency vs. homeogeneity
(Cortina, 1993; Schmidt, 1996)
internal consistency- refers to the degree of interrelatedness among items
homogeneity- refers to unidimensionality
- internal consistency is a necessary but not sufficient condition for homogeneity
Scale length and Alpha
- the higher the # of items, the higher the alpha– even if the scale is not unimdensional
- alpha is essentially a function of the number of items in the scale and it shoul dbe interpreted with the number of items in mind
- if a scale has more than 14 items, its very hard to have an alpha lower than .7
(Cortina, 1993)
What are the assumptions of Classical Test Theory?
CTT assumes ZERO. 1) The mean of the error scores is zero. 2) The correlation between True Score and Error Score is zero. 3) The correlations between error scores is zero.
What is our goal when we use CTT to assess reliability?
To separate 1) the stable characteristics of the individual (true score) from 2) the inconsistent factors (error)
Reliability (CTT)
2 interchangeable definitions: The consistency of the measure. How much the measure is free of error.
In CTT, if a source of variance is the same across test-takers, it is…
Systematic variance, which is not treated as error.
3 Types of Reliability Methods (CTT)
1) Test Retest (same test, taken twice) 2) Alternate forms (2 tests) 3) Internal Consistency (split half or Cronbach’s alpha)
Reliability Index (CTT)
Correlation between observed and true score
Reliability Coefficient (CTT)
Correlation between parallel measures
Lumsden (1976)
Lays out all the issues/criticisms with Classical Test Theory (remember the flogging wall?) - Tests are unreliable - All other things equal, longer tests are better up until boredom or fatigue - Higher item covariances are better, up until attenuation paradox could occur
What are the 6 categories of sources of variance in CTT?
1) Lasting & general (reading comprehension) 2) Lasting & specific (ability level) 3) Temporary & general (health) 4) Temporary & specific (test taking strategy) 5) Systematic issues (time limits) 6) Random events (lucky guesses)
Cronbach’s Alpha
The mean of all split-half correlations
Attenuation Paradox
In CTT, reliability and validity increase together, but after a certain point any further increases in reliability will decrease validity
Sampling distribution
The distribution of a statistic gathered from all possible samples of the population. From the central limit theorem, we know that the sampling distribution will always be normal.
Sampling error
The difference between the sample statistic and the population statistic, caused by sample characteristics differing from the population. Can be corrected with a larger sample size or a more representative sample.
Content Validity
The degree to which the measure covers a representative sample of the content. General steps: 1. define content domain; 2. Select SME’s; 3. Provide framework for rating or matching items to the content domain; 4. Collect & summarize data
Criterion-related validity
Correlation (Validity Coefficienct; r xy ) between predictor (IV) and criterion (DV). Can be Concurrent (IV & DV collected simultaneously) or Predictive (DV collected after IV).
Construct validity
Accuracy of inferences (convergent & discriminant).
Multitrain-miultimethod matrix (MTMM)
A matrix of multiple meathods used to measure multiple traits; Same construct, diff. method correlations (Monotrait-heteromethod; Convergent) should be higher than diff. constructs, same methods (Heterotrait-monomethod)
Restriction of Range
Removal of portion of sample (e.g. cutoffs) often results in attenuation.
Attenuation
decrease in validity coefficient; Caused by unreliability and restriction of range; Statistical corrections possible
Validity coefficient
predictor-criterion correlation
Common problems in Criterion-related validation
Small sample size; Low reliability (pred. & crit.); Restriction of range; The criterion problem
The Criterion Problem
Contamination or deficiency in our criterion.
Validity
Validity is a representation of the scientific confidence in the inferences and information from a test.
Cohen (1983)
Talks about the cost of dichotomization, which is that is reduces the correlation (r), which then reduces 1) effect size 2) the value of the t-test 3) statistical power
Cox (1980)
This is the paper that tried to find the optimal # of scale response options. He found that a) 7 +/-2 is best for keeping the responses meaningful to the respondent, and b) 3 to 9 response options are best for determining differences among people.
Tourangeau et al (2000)
The authors line out the psychology of survey-takers, the “Response Process Model”, saying that responses come from the process of 1) comprehension 2) retrieval 3) judgement 4) response
Standard Error of Measurment (CTT)
the standard deviation for an person’s expected score across a large number of equivalent tests
MacCallum et al (1999)
Says that there is not rule of thumb for sample sizes of EFA’s. It needs to be determined by 1) Sample size 2) Communality of the items 3) Overdetermination of the factors The lower your communality, the higher the sample size needs to be.
Preacher & MacCallum (2003)
The article that warns people not to use “little jiffy” when doing an EFA analysis because it uses a PCA (bad: items load on factors; assumes correlation) and a Varimax rotation (bad: assumes variables are unrelated).
Communality of the Item
How much the item varies (%) due to latent factors. More communality means less random error. Less error means you don’t need a big sample size to estimate factor loadings.
Overfactor
Retaining too many factors in an EFA, as opposed to underfactoring (retaining too few). Better to overfactor than underfactor.
Unified Framework of Vaidity (definition)
A visualization of the important inferences in validation that describe relationships between predictor and criterion, measure and construct domains.
Binning & Barrett (1989)
Point 1: Validity is confidence in inferences and information from a test and not a characteristic of the test itself, and validation is a reflection of the process of developing scientific theories. Point 2: Provide unified framework of validity
Method Effects
The method is causing higher correlations; detected in the difference in heterotrait-monomethod and corresponding heterotrait-heteromethod matrices.
Campbell & Fiske’s (1959)
4 Rules: 1) Validity diagonal correlations should be statistically significant & practically meaningful; 2) Validity diagonal element should be larger than others in the same row and column in the heterotrait-heteromethod triangles. 3) Validity diagonal element should be larger than others involving that trait in the heterotrait-monomethod triangles. 4) Pattern of correlations among traits should be the same in all heterotrait triangles
Measurement
Application of qunatitaive values to behavioral samples Be refined enough to allow maximal transmission of information, but not too nuanced as to encourage measurement error.
Internal Validity
The degree of correctness (truth, value, legitimacy) of inferences about the causal connections between focal constructs (the inference that x causes y).
Item Difficulty in CTT
The proportion of test takers who endorse/get the item correct for dichotomous items, or the item’s mean for items that are polytomous.
Item Discrimination in CTT
How well an item does at differentiating among test takers who have different levels of the trait being measured For CTT, Calculated by correlating the score on a particular item with total score on the scale (positive and .3 or greater seems to be the common heuristic)
Cohen’s D
The amount of difference between 2 groups in standard deviation units
Do’s and Don’t of Field Experiments in Orgs

SEM Model Example

Construct Validity

Endogenous vs. exogenous variables
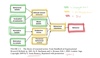
Zickar 2020 Main Points

Measure Development Steps
according to Zickar 2020

Advantages and Disadvantages of
CTT
EFA
CFA
IRT
Zickar (2020)

Unified Validity
THE figure
Binning & Barrett 1989

Questionable Research and Analyses Practices
(Campbell & Wilmont, 2018)

Why null hypothesis significance testing is problematic
Campbell & Wilmont (2018)

Positives of Theory
Campbell & Wilmont, 2018

Steps of Theory Construction
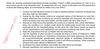
What theory is not

4 Key ingredients to a theory
(Campbell & Wilmont, 2018)
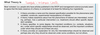
Meditation-product of coefficients approach
Uses the coefficients of the a and b paths to estimate the indirect path
- Sobel test to assess significance
- Preacher/Hayes Macro using bootstrapping- less conversative
(MacKinnon et al., 2010)
Mediation- difference in coefficients approach
Estimates the effect by taking c-c’
aka the direct effect without mediators-direct effect with mediators to estimate the side
takes that and divides by standard error to determine significance
Moderated meditation testing
Run regressions or SEMs for the two groups and see if there is a difference in parameters/fit.
(MacKinnon et al., 2007)
3 step process to moderation
(Chan, 2011)
- Enter X and Z as predictors in block 1
- Enter XZ as predictor in block 2
- See whether there is a statistically significant increase in variance accounted for in the criterion
- if significant, plot the simple slopes to see the nature of the interaction
Benefits of ESM
(Beal, 2015)
Reduction of memory biases
reduction of method biases
Ease of collection/implementation
Key attributes of ESM
(Beal, 2015)

Goal of ESM
To capture a representative range of experiences as they occur in their natural setting/environment (Beal, 2015)
Issues with ESM
- Participant burnout or fatigue- careless responding, reduced response rates
- Responders can anticipate the next survey questions, which may influence their responses
- measurement equivalence- is the measure equivalent over each repeated measure
- missing data is inevitable
- internal validity issues– because of the repeated measures it can be hard to tell which caused which