Critical Numbers Flashcards
What are the target and sample populations?
- Target population = larger population
- Sample population = sub-set of target population as we can’t sample everyone
What are the 4 main types of bias?
- Sampling bias = individuals more/less likely to be included
- Recall bias = cannot remember specifics
- Social-desirability bias = study group tell us incorrect information due to societal pressure
- Information bias = measurement error
What are the 3 types of study designs?
- experimental (researcher changed something) vs. observational (researcher has not intervened, just observed)
- retrospective (look back into past, subject to bias) vs. prospective (collect information at start and follow up over time)
- individual vs. population
What is a case-control study? What are its strengths and weaknesses?
- Find people with a disease, look back in time and see whether they were exposed to risk factor in question.
- Retrospective
Positives
- Works well for investigating rare outcomes
- Relatively fast/cheap (no follow up)
- Few ethical considerations
Negatives
- Cannot prove causation/eliminate confounders
- Can be difficult to establish order of events
- Possibilities for bias (recall bias)
- Can only investigate a single disease
What is a cross-sectional study? What are its strengths and weaknesses?
•Take a sample, see who has the disease right then and there
Positives
- Relatively fast/cheap (no follow up)
- Few ethical considerations
- Generates hypotheses
Negatives
- Cannot prove causation/eliminate confounders
- Less suitable for rare disease
- Difficult to get an understanding of order of events
- Sample bias (when you do the study)
What is a cohort study? What are its weaknesses?
- Collect information from a sample, some with exposure, some do not (none should have the outcome).
- Follow-up over time and see if there is a link between exposure and outcome
- Prospective
Positives
- Few ethical considerations
- Clarity on event sequence
Negatives
- Cannot prove causation/eliminate confounders
- Not suitable for rare disease or when disease takes a long time to develop
- Time consuming/expensive
- Difficulty following up
- Patients can change behaviours in the cohort
What is randomised controlled trial (RCT)? What are its strengths and weaknesses?
- Multiple groups (referred to as arms), give each different exposures and compare outcomes.
- We can balance arms by matching, randomising, cross-over, placebos, blinding
Positives
- Considered gold standard, can prove causation by eliminating confounders…
- Particularly with extensions (cross-over trial)
- Random (less bias)
Negatives
- Not suitable for rare outcome or when outcome takes a long time to develop
- Time consuming/expensive
- Often unethical
- Issues with people following up, compliance etc.
What is a crossover trial? What are its weaknesses?
- Extension of RCT. Everyone has all arms of trial
- Weaknesses = more technical analyses, not always suitable (even if standard RCT is)
What is an ecological study? What are its strengths and weaknesses?
- Massive sample (if not whole population) by looking at data previously collected to look at prevalence, trends and correlation
- Look at populations, not individuals
Positives
- Fast/cheap
- Very large sample (small standard error)
- Easy to do
- Good first step to generate hypothesis
Negatives
- You do not know how data was collected (variation/bias)
- Often absent/inconsistent/incorrect data – variation in diagnosing criteria
- Cannot prove causation
Ecological fallacy – where there is a correlation between predictor and outcome, but this does not mean causation.
Explain what the ecological fallacy is.
The ecological fallacy occurs when you make conclusions about individuals based only on analyses of group data. Just because two things are linked, it doesn’t imply a causal relationship
What is a sample? What is the difference between the target population and the sample population?
•A sample is a group we are using to represent the population.
–Target population – the population the sample represents
–Sample population – the people whom data is collected
The sample population should generalise the target population.
What are the five main types of sampling?
- Random sampling - random number generator, “draw a name out of a hat”. Usually preferred way of sampling
- Systematic sampling - count of the list and every “k”th element is taken
- Convenience sampling - The first people who approach you are used. Easiest technique but likely the worst
- Cluster sampling:
●Divide the population into groups, usually geographically
●Each group is called a cluster, or block
●Clusters are randomly selected, each element in the selected cluster used
- Stratified sampling:
●Divide the population into groups/strata, based not on geography, but some characteristic, e.g. males and females
●A sample is taken from each of these strata using either random, systematic or convenience sampling
What are the 6 main types of bias?
- Sampling bias – sample is not representative of the target population (encompasses other forms of bias)
- Recall bias – people fail to remember specifics innocently
- Social-desirability bias – incorrect information is given due to societal pressure
- Information bias – where data is consistently measured wrong (may be referred to as observation bias)
- Volunteer bias – volunteers for a study often aren’t representative
- Produced bias – subjects in different arms are treated differently
What are 3 other forms of bias that are related to screening?
•Selection bias - people who sign up for screening programmes are not representative of the whole population (higher/lower risk)
–E.g. women in higher socioeconomic groups more likely to attend cervical cancer screening, who are at lower risk
•Lead time bias - screening improves survival length?
–Maybe no, just detected illness sooner
•Length-time bias - screening improves survival length?
–Maybe no, people who survive longer are more likely to be picked up by screening
What is a confounding factor?
- A confounding factor has to be related to the outcome, and the characteristic of interest (exposure)
- Examples:
- There is a high rate of lung cancer among those who have breath mints. Confounder is smoking.
- There are high rates of cancer among people in care homes. Confounder is old age.

Which type of study design below would be best to investigate the following;
“Identify patients who have had previous MIs and compare their diet, smoking habits and exercise activity with people that are similar but have not suffered previous MI?”
A. Ecological study
B. Cohort study
C. Cross sectional study
D. Longitudinal study
E. Case control study
Answer: E, take the group of individuals who have had heart attacks and look back in time to investigate diet smoking habits and exercise, then do the same for the group who have not suffered heart attacks
A research group wants to estimate the UK national prevalence of coeliac disease. What study design would be most appropriate?
A. Cohort
B. Randomised control trial
C. Cross sectional
D. Longitudinal
E. Case control
Answer: C – Cross sectional, gives a snapshot of prevalence with no reference to time
To calculate the average 100m sprint time, a research group advertises they are looking for participants to run around a track and select participants by a convenience sample.
Which type of bias is this sampling method subject to?
A. Volunteer bias
B. Recall bias
C. Lead time bias
D. Misclassification bias
Answer: A – people who enjoy exercise are more likely to volunteer for the study than those who are not, thus the sample is not entirely representative
To draw a sample from primary school children, researchers line children up and count off the children 1, 2, 3, 1, 2, 3… placing them into 3 different groups
Which term best describes this form of sampling?
A. Random sampling
B. Systematic sampling
C. Convenience sampling
D. Cluster sampling
E. Stratified sampling
B. Count of the list and every “k”th element is taken, here K is 3
A study is designed to examine the relationship between blood pressure (BP) and occupation group. If age is a confounder, then:
- A. Age is linked to diet, and diet affects BP
- B. Different occupations will have different ages, BP will change with age
- C. Younger and older people have the same occupations
- D. Different occupations have similar age groups
B
Which of the following is true of observational studies?
- A. They can have retrospective studies
- B. They are always shorter than an experimental study
- C. They are more powerful than experimental studies
- D. Participants must be randomised into groups before analysing the data
A
University students with insomnia were randomly assigned with a simple randomisation (flip of a coin) to receive either CBT or usual care. This randomisation method ensures:
- A. Each student has an equal chance of being in any treatment group
- B. The student is unaware of the treatment group to which they are assigned
- C. The same number of students will be allocated to each group
- D. Although individuals receive different treatments, each student will be allocated to the treatment most likely to benefit them
A
Sampling bias occurs when:
- A. Certain individuals are more likely to be included in the study than others
- B. 40% of the original sample drop-out of the study at random
- C. Observational studies are used instead of RCTs
- D. The researcher is not blinded within the study
A
In a cohort study:
- A. It is possible to look at a range of outcomes
- B. We use a snapshot of time
- C. We can examine very rare diseases
- D. We don’t worry about confounding
A




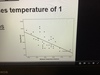
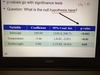
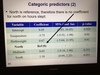












