Statistics Flashcards
population
set of all individuals of interest in a study population = parameter
parameter
numerical value that describes a population can be a single measurement or set of measurements
sample
set of individuals selected from a population, representative of population in a study sample = statistic
statistics
numerical value that describes a sample can be a single measurement or set of measurements
descriptive statistic
statistical procedures that are used to summarize, organize, simplify data - make raw score meaningful e.g. mean, median, mode
inferential statistics
techniques that allow us to study samples then make generalizations about the population - infer sample -> population
sampling error
discrepancy/ amount of error that exists between a sample statistic and population parameter - important to consider in inferential statistics
construct
internal attributes/ characteristics that cannot be directly observed but are useful for describing and explaining behavior - hypothetical e.g happiness
operational definition
defines construct in terms of observable behaviors e.g. intelligence defines as performance on IQ test
nominal scale
categorical organization - can only measure qualitative difference e.g gender, country of origin, hair color
ordinal scale
categories organized in a certain sequence, differences are quantitative - amount between one person and next is not consistent e.g. class rank, rating scale
interval scale
ordered categories that are intervals of exactly same size with an arbitrary zero point - 0 does not mean the absence of the construct being measured e.g. celsius scale, temp
ratio scale
interval scale with absolute zero point - can describe differences between categories in terms of ratios (one thing is 3 times larger than another) e.g. weight, height, speed
discrete variables
separate, indivisible categories - whole numbers or specific categories - no decimals e.g 3 goals scores
continuous variables
infinite number of possible values that fall between any two observed values - divisible into infinite number of fractional parts e.g. height
real limits
boundaries of intervals for scores that are represented on a continuous number line - each score has two limits, half way between scores (upper real limit, lower real limit) e.g. if you have observed value of 8, actually represents range from 7.5 - 8.5 (kind of like rounding)
correlational method
two variables observed to see if there is a relationship between the two
experimental method
establishes cause and effect relationship between variables - must manipulate one variable, observe second - controlled research situation
non-experimental method
variable determines group (those that have depression) - don’t manipulate
independent variable
manipulated variable - 2+ treatment conditions
dependent variable
observed for changes to assess effect
control
does not receive manipulated experimental treatment, baseline for comparison
quasi-independent variable
groups not created by manipulating independent variable - participent variable (male/female) - time variable (before/after)
summation notation
a way to represent scores n ∑ xi i = 1 i = the starting point of the scores n = the stopping point

µ
population mean
x
sample mean
σ
population standard deviation
s
sample standard deviation
σ2
population variance
s2
sample variance
SS/n (df w/ sample)
P
population portion that have particular attributes
p
sample proportion that have particular attributes
ρ
population correlation coefficient
r
sample correlation coefficient
N
population number of elements
n
sample number of elements
H0
null hypothesis
H1
alternative hypothesis
α
alpha probability of a type 1 error
B
beta probability of a type 2 error
type 1 error
incorrect rejection of a null hypothesis
false positive
thinking there is an effect when there isnt
type 2 error
incorrectly retaining a false null
fals negative
thinking there isnt an effect when there is one
frequency distribution
organized tabulation of the number of individual scores located in each category on the scale of measurement - takes disorganized scores and placed them in order from highest to lowest - see entire set of scores at glance - categories based odd measurement scale - can be graph or table
grouped frequency distribution
when the data covers a wide range of values and it is unrealistic to list individual scores - rule 1: ~10 class intervals - rule 2: relatively simple width (2, 5, 10) - rule 3: interval starts with a score that is multiple of the width - rule 4: all intervals should be the same width
bar graph
uses horizontal or vertical bars to show comparisons among categories - nominal/ordinal
ogive
curve of the cumulative frequency distribution or cumulative related frequency distribution - express simple frequency as percentage of total frequency - cumulate and plot these percentages (e.g. lowest scores makes up 5%, next score makes up 6% but the cumulative frequency is 11% so that is what is plotted for score 2)

polygon
a line drawn to join all the midpoints of the top bars of a histogram - like an ogive, but does not use cumulative frequencies or smooth lines - to convert to ogive, add up percentages before each bar

histogram
an area diagram -> bars portray frequencies of possible values of a variable - continuous variables (this is why the bars touch) - set of rectangles along the intervals between class boundaries - areas proportional to the frequencies in corresponding classes
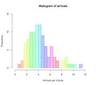
population distributions
cant find absolute frequency but can find relative frequencies e.g. don’t know how many fish encompass the population in a lake -> don’t know how many trout or salmon, after research can say that there are twice as many trout as salmon
percentile
score point below which a specified % of the scores in a distribution fall
- compute the percent * N
- round this figure so that it ends in .0 or .5 whichever is closer
- if rounded value ends in .5 the desired centile is the next higher value, if ending in .0 split the difference with the next higher score
percentile rank
precent of cases which are below a specific point in the distribution
- write down exact limits of the interval which contain the score whose rank is to be obtained
- interpolate between the cumulative percents to dind desired CR
exact limit/ cum %
Y/A
X/B
Z/C
X-Z/Y-Z = B-C/A-C