M1 U1 - Problem Identification - Q1 Flashcards
Interesting fact from Gartners about DS projects in industry
A Gartner study showed that 85% of data science projects fall short of expectations.
Reasons for DS project failure (5)
- Insufficient or inappropriate data.
- Lack of technical data science skills.
- Issues with project management .
- Inaccurate interpretation of results.
- Mismanaged client expectations .
Steps of a DS project
- Understand business need
- Identify business objectives (to address the business need)
- Evaluate whether data science methods can be used to facilitate the company’s efforts to meet them
- Develop a solution vision
- Develop set of analytic objectives based on solution vision (i.e. making the solution vision more specific)
Describe an analytic objective
Will support a business objective and states how analytical methods can be applied to a scenario to meet a business objective.
What is the Evidence Value Proposition (EVP) framework?
Five-step framework developed to help determine the desired analytic objectives and provide evidence that the objectives can be met with the appropriate data and technology.
List the steps of the EVP framework
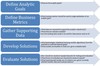
Describe a business objective
A business objective has a result attached to it, to make it measurable
Since when has AI been applied commercially?
- Rules-based systems have been around at least since the 1980s
- Modern AI (uses statistical ML techniques) has been around since the 90’s
What is “bespoke AI”?
An AI solution that is more or less tailor-made for the customer.
What is the AI hypothesis

What is data science readiness?
Two Steps (heavily paraphrases):
- Do they have good enough data to do DS with? -> they’re ready for DS
- Is there a DS solution that is practically and economically feasible? -> DS is ready for them

What are the milestones of AI maturity?
Data Science Ready. The organization has identified all the current sources of data to be considered, and those data have been normalized and integrated into a form that supports data science (statistical analysis, correlation, prediction, etc.). At this point the organization is ready to begin an engagement with data scientists and AI developers. The Case in point scenario showed that Monro Inc. was data science ready. During interviews with the information technology team, the data manager provided the adequate information about the company’s data architecture, appropriate data sources that would support the MotoManager app, as well as data that would be useful to build the desired prediction model.
Data Science Enabled. Preliminary analysis indicates that the data support the desired analytic objectives (useful correlations are identified, predictive models prove to be accurate enough, etc.). At this point, the organization can claim that it is ready to use data science to influence the capabilities of its workflows, products and services.
AI Ready. The organization has determined how to leverage the insights from data science (e.g. predicting customer preference) as part of an operational process, and has implemented the appropriate software or software extensions to integrate data science into a relevant workflow, product or service. At this point, the organization can claim that it understands how to incorporate AI into its workflows, products and services to provide enhanced capabilities for end users.
AI Enabled. The organization has deployed the new software in a relevant context, and is able to directly measure the impact (e.g., increased sales). At this point the organization can claim that it has implemented AI, and is gathering feedback to show that it really works with real end users. An organization can introduce the data science decision into a real world setting and measure if this implementation works.

List a key implication for each AI maturity stage
Data Science Ready. At this point the organization is ready to begin an engagement with data scientists and AI developers.
Data Science Enabled. At this point, the organization can claim that it is ready to use data science to influence the capabilities of its workflows, products and services.
AI Ready. At this point, the organization can claim that it understands how to incorporate AI into its workflows, products and services to provide enhanced capabilities for end users.
AI Enabled. At this point the organization can claim that it has implemented AI, and is gathering feedback to show that it really works with real end users.