L25 - Distributed Lags Flashcards
What does a scatterplot look like for a binary dependent variable?
- e.g. say if Yi = {0,1}
- Then the points can only lie on the x-axis (y=0) or the line y=1 –> this makes it very hard to fit a linear equation to the scatterplot
- So instead of fitting a linear line, we fit a logistic curve with a sigmoid shape

How can you interpret the coefficients in limited dependent variable models?
- What is the expected value of Y|X?
- Well if Y can only be 0 or 1 (binary) then its expected value must be ) and 1 multiplied by the probability of occurring ( which will just leave whatever is multiplied by 1)
- The F(X) is the conditional probability of getting Y=1
- The first derivative gives the marginal effect of a change in X on the expected value of Y –> NOT CONSTANT
- F’(X) = β * Pr(Y=1) * Pr(Y=0)
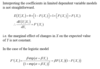
At what point do we evaluate a limited dependent variable model?
- at the mean of the data (use X(bar) in the calculation for the marginal effect)
Can other functions be used to solve Limited Dependant variable models?

What are Distributed Lags?
Distributed lags are dynamic relationships in which the effects of changes in some variable X on some other variable Y are spread through time.
Distributed lags can arise for a variety of reasons including:
- Costs of adjustment –> costly to change Y variable all at once
- The effects of expectations –> take a while for expectations to adjust
Below depicts two ways we can account for them in a regression

How do econometrician account for correlation between lagged X variable when using a Infinite distributed lag model?
- With geometrically declining weights, the future we go into the past the less of an impact its weight will have thus beta for each coefficient is halved each time
- It could be said that βi = β0λi and as long as λ < 1 (so it converges), will tend to zero –> the weights get less and less the further in the pas the lag is
- this reduces the number of parameters we need to estimate from an infinite number of β’s to just two: β0 and λ
- both have the same marginal effect –> the geometric sum of all the β in the infinite model = 1
*

How can you estimate a geometrically declining weifghts model?

What is the adaptive expectation model?

What is the problem with the adaptive expectations model?
- it is persistently and predictably wrong
- it is systematic below actual inflation
