Reading 12 - Multiple Regression and Issues in Regression Analysis Flashcards
Describe what Multiple Regression is ….
Is regression analysis with more than one independent variable. It is used to quantify the influence of two or more independent variables on a dependent variable.
What does the Residual represent in a regression equation?
Is the difference between the observed value Yiand the predicted value from the regression
What is the intercept term in a multiple regression equation?
Is the value of the dependent variable when the independent variables are all equal to 0
What is the formula to calculate the t-statistic in a multiple regression equation?
It is ued to test the significance of the individual coefficients in a multiple regression

How many degress of freedom does the t-statistic have in a multiple regression?
n-k-1
k= the # of regression coefficients in the regression
What are we doing when we test for stastical significance?
It means testing the null hypothesis that the coefficient is zero vs the alternative that it is not
What is the p-value?
The smallest level of significance for which the null hypothesis can be rejected.
What determines whether we can or cannot reject the hypothesis when comparing p-values to the significance level?
- if the p-value is less than the significance level, the null hypothesis can be rejected
- If the p-value is greater than the significance level, the null hypothesis cannot be rejected
Using this data, test the null hypothesis that PR is equal to 0.20 vs the alternative that it is not equal to 0.20, using a 5% significance level.
The t-value is 2.02 from the back of the book.
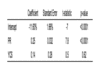

What does a confidence interval look like?

What does a 10% significance level mean?
Is the same thing as a 90% confidence level
What are the assumptions made with the multiple regressions regarding the model’s standard error?
- a linear relationship exists between the independent and independent variables
- the independent variables are not random, and there is no exact linear relation between any two or more independent variables
- the expected value of the error term, conditional on the independent variable, is 0
- the variance of error terms is constant for all observations
- the error term is normally distributed
What is the F-statistic?
Is used to test whether at least one of the independent variables explains a significant portion of the variation of the dependent variable
Is an f- statistic a one tailed or two tailed test?
Always one - tailed test
How is the f - statistic calculated?

What is the decision rule for an f - test?
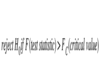
An analyst runs a regression of monthly value-stock returns on five independent variables over 60 months. The total sum of squares is 460, and the sum of squared errors is 170.
Test the null hypothesis at the 5% significance level that all five of the independent variables are equal to zero.
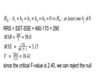
What does an R2 of 0.63 indicate about a model?
that the model, as a whole, explains 63% of the variation in the dependent variable
How is the R2 calcuated?

Why is the R2 not considered a reliable measure of the explanatory power of the regression model?
because the R2 almost always increases as variables are added to the model, even if the marginal contribution of the new variables is not statistically significant
To overcome the deficits of the R2, statisticians have created an adjusted R2. What is the formula for this equation?

What are dummy variables?
occassions when the independent variables are binary —–it is either “on” or “off”
Dummy variables are assigned a 0 or 1 only
What are the three primary assumption violations that you encounter doing multiple regressions?
- Heteroskedasticity
- serial correlation (ie autocorrelation)
- multicollinearity
What is heteroskedasticity?
occurs when the variance of the residuals is not the same across all observations in the sample
This happens when there are subsamples that are more spread out than the rest of the sample.
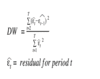
