Basic Tools Flashcards
(36 cards)
Describe the properties of Linear Functions.
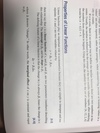
Example 1 - Linear Housing Expenditure Function
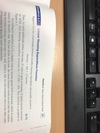
Example 1 - part 2

Example 1 - part 2
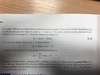
Example 2 - Demand for CD’s

Let grthemp denote the proportionate growth in employment, at the county level, from 1990 to 1995, and let salestax denote the county sales tax rate, stated as a proportion. Intrepret the intercept and slope in the equation:
grthemp = .043 - .78 salestax
According to the equation, the constant .043 is the proportionate growth in employment where there are no taxes on sales. In other words, if there are not taxes on sales, the employment would grow by 4.3 percentage points.
The slope can be interpreted as follows: an increase (decrease) of the sales tax rates by one percentage point decreases (increases) the growth in employment by -0.78 percentages points.
Suppose that in a particular state a standardized test is given to all graduating seniors. Let score denote a student’s score on the test. Someone discovers that performance on the test is related to the size of the student’s graduating high school class. The relationship is quadratic:
score = 45.6 + .082 class - .000147 class2
where class is the number of students in the graduating class.
1) How do you literally interpret the value 45.6 in the equation? By itself, is it of much interest? Explain.
The value 45.6 is the intercept in the equation, so it literally means that if class = 0 then the score is 45.6. Of course, class = 0 can never happen. So, by itself, 45.6 is not of much interest. But it must be accounted for to use the equation to obtain score for sensible values of class.
Suppose that in a particular state a standardized test is given to all graduating seniors. Let score denote a student’s score on the test. Someone discovers that performance on the test is related to the size of the student’s graduating high school class. The relationship is quadratic:
score = 45.6 + .082 class - .000147 class2
where class is the number of students in the graduating class.
2) From the equation, what is the optimal size of the graduating class (the size that maximizes the test score)? (Round your answer to the nearest integer.) What is the highest achievable test score?
Compute the first derivative of the function score with respect to the variable class, and set it equal to zero. Solving the equation for the variable class we get
class = .082/ (2 X 0.000147) = 278.91. The optimal number of students is 279. The largest achievable score is 45.6 + (.082 X 279) - [.000147 X (279)2] = 57.04
Suppose that in a particular state a standardized test is given to all graduating seniors. Let score denote a student’s score on the test. Someone discovers that performance on the test is related to the size of the student’s graduating high school class. The relationship is quadratic:
score = 45.6 + .082 class - .000147 class2
where class is the number of students in the graduating class.
3) Does it seem likely that score and class would have a deterministic relationship? That is, is it realistic to think that once you know the size of a student’s graduating class you know, with certainty, his or her test score? Explain.
Of course it is not at all realistic to think that a student’s score is determined only by the size of his or her graduating class. This would imply that all students graduating in the same year from the same high school would have the same score. The class size could affect the learning experience, but most likely in a very marginal way or not at all.
It is hard to believe that a deterministic relationship with any set of variables exists, because the score might be affected by plenty of factors that cannotbe accounted for, as intelligence, personal situation, interest for the subject, physical and emotional conditions during the test etc
Suppose that person A earns £35,000 per year and person B earns £42,000.
1) Find the exact percentage by which person B’s salary exceeds person A’s.
42,000−35,000 / 35,000
= 0.2
so B earns 20% more than A
Suppose that person A earns £35,000 per year and person B earns £42,000.
2) Now, use the difference in natural logs to find the approximate percentage difference.
Let log(·) denotes the natural logarithm, then
log(42,000)−log(35,000) = 0.1823
The approximate percentage difference is 18%
What is the meaning of ceteris paribus?
When using ceteris paribus in economics, one assumes that all other variables except those under immediate consideration are held constant. For example, it can be predicted that if the price of beef increases—ceteris paribus—the quantity of beef demanded by buyers will decrease.
What is the meaning of a causal effect?
Therefore, causal effect means that something has happened, or is happening, based on something that has occurred or is occurring. A simple way to remember the meaning of causal effect is: B happened because of A, and the outcome of B is strong or weak depending how much of or how well A worked.
Define cross sectional data.
Cross-sectional data are observations that come from different individuals or groups at a single point in time. If one considered the closing prices of a group of 20 different tech stocks on December 15, 1986, this would be an example of cross-sectional data
Define Data frequency.
The frequency of a particular data value is the number of times the data value occurs. For example, if four students have a score of 80 in mathematics, and then the score of 80 is said to have a frequencyof 4. The frequency of a data value is often represented by f.
Define non experimental data
Non-experimental research is the label given to a study when a researcher cannot control, manipulate or alter the predictor variable or subjects, but instead, relies on interpretation, observation ( aka observational data) or interactions to come to a conclusion
Define panel data
panel data or longitudinal data are multi-dimensional datainvolving measurements over time. Panel datacontain observations of multiple phenomena obtained over multiple time periods for the same firms or individuals
define pooled cross section
A data configuration where independant cross sections, usually collected at different points in time, are combined to produce a single data set.
Define time series data
A time series is a series of data points indexed in time order. Most commonly, a time series is a sequence taken at successive equally spaced points in time. Thus it is a sequence of discrete-time data.
What are logirithms and natural logirithms?
Logarithms are like the opposite of exponents. Just like how adding and subtracting are “opposite” operations (and multiply/divide). For example:
103 = 1000. 10 is the base, 3 is the exponent, 1000 is the result.
The opposite direction of this is:
log10(1000) = 3. Again 10 is the base (it should be subscripted), 1000 is the result, and 3 is the exponent. Except now you’re working backwards from the result.
Generally log() means base 10. If it’s not base 10, they’ll write what base it is in subscript. Natural log, or ln(), is a special log where the base is e.
Why do we use logirithms?
There are two main reasons to use logarithmic scales in charts and graphs.
The first is to respond to skewness towards large values; i.e., cases in which one or a few points are much larger than the bulk of the data.
The second is to show percent change or multiplicative factors
Coefficient of determination.
The coefficient of determination, R2, is used to analyze how differences in one variable can be explained by a difference in a second variable. For example, the timing of when a person gets pregnant has a direct relation to when they give birth.
More specifically, R-squared gives you the percentage variation in y explained by x-variables. The range is 0 to 1 (i.e. 0% to 100% of the variation in y can be explained by the x-variables.
Control variable
Controlled variables are quantities that a scientist wants to remain constant, and she or he must observe them as carefully as the dependent variable
Covariate
Is the same as an explanatory variable/independant variable.
It is a variable that is used to explain variation in the dependant variable.